Full screen
Share
Show pages
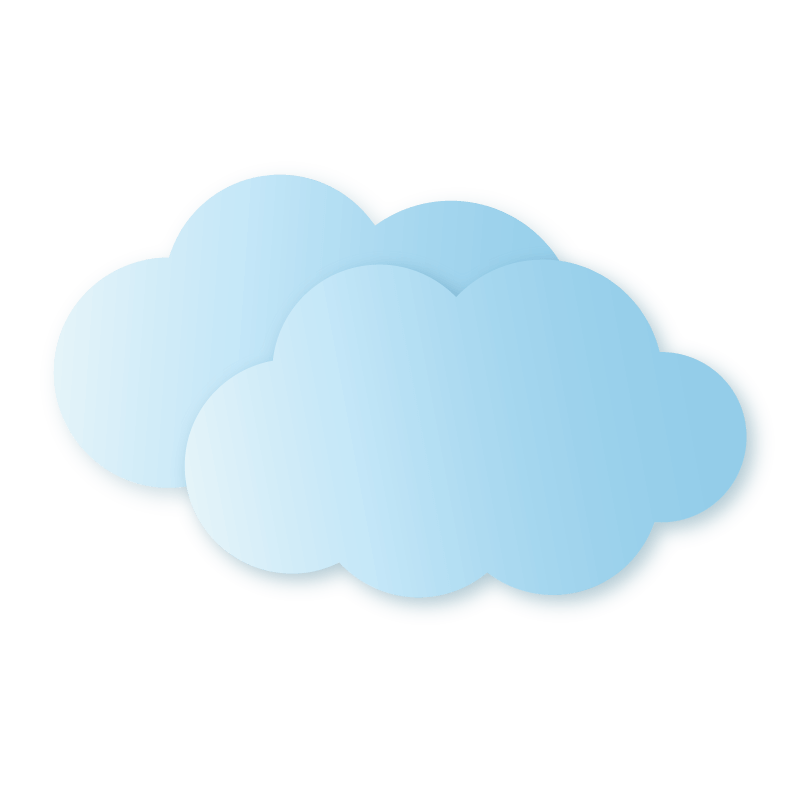
AI Generated Text
Ryan Morrison
Created on November 16, 2022
Over 30 million people build interactive content in Genially.
Check out what others have designed:
SLYCE DECK
Personalized
LET’S GO TO LONDON!
Personalized
ENERGY KEY ACHIEVEMENTS
Personalized
HUMAN AND SOCIAL DEVELOPMENT KEY
Personalized
CULTURAL HERITAGE AND ART KEY ACHIEVEMENTS
Personalized
DOWNFALLL OF ARAB RULE IN AL-ANDALUS
Personalized
ABOUT THE EEA GRANTS AND NORWAY
Personalized
Transcript
Non-existent Reference page entries
Inability to refer to post-2019 events accurately
Self-contradictory statements
Innacurate claims presented as fact
Conventional themes and patterns of journalistic and academic rhetoric
Innaccurate details in summarizing
Topical Drift
Fictitious People and Instances
Click the pins to see explanations and links.
Click to see what can not be identified as AI generated
How to identify AI generated text
STEMware Inc.
Because of the creative nature and types of academic sources used in LLMs like GPT-3, they can easily create the patterns of reference pages when prompted, but not an accurate working reference entry. Click here for a demonstration.
Sometimes, text will appear self-contradictory. Depending on the temperature setting of GPT-3, for example, so-called 'randomness' can be increased or decreased. On the low end, text will become repetitious of the input -- this functions well for summarizing and paraphrasing. However, when the temperature is increased, the algorithm becomes more creative and random. So, logic and focused argumentation can quickly dissipate. Click here to access a basic GPT-3 text generating platform, with temperature adjustment tools (log in required). ChatGPT Update: This happens much less frequently as ChatGPT is significantly better trained and has preset parameters that preclude the problems of temperature settings.
The source text of LLMs is static, i.e. events that happened after the creation of the language model in question are not included. Since GPT-3's source text was created in 2019, prompts that focus on post-2019 topics do not elicit focused responses. OpenAI claims that it is post 2021, but my experience was that the more recent the topic, the more difficult it was for GPT-3 to generate accurate information. Click to learn more about the source text of GPT-3. On page 2, there is a great visualization of the source text 'The Pile'.
One of the early criticisms of GPT-3 and LLMs focused on the potential to automate the creation of racist, sexist, homophobic and otherwise problematic text. Since the source text is so vast, the inclusion of sources with problematic content was inevitable. As a result, OpenAI and others added filters to negate the likelihood that their algorithms would generate this text. As a result of these filters and other parameters, when the content becomes too close to a filtered or unfamiliar topic, it will 'drift' into more comfortable territory. The seminal paper examining this and other social issues surrounding LLMs was "On the Dangers of Stochastic Parrots: Can Language Models Be Too Big?" by Bender, Gerbu, McMillan-Major & Shmitchell Click here to read the full text
Parallel to the issues of topical drift, common themes and approaches to topics which may be considered cliché often appear. For example, from a Canadian perspective, when comparing common social programs like medicine, most often the comparison will be to that of the United States. Therefore, if posed with an unfamiliar or unconventional comparison -- perhaps the medical system of Tanzania -- the generated text will not be accurate and will often resort to comparisons where the corpus of the source text is more robust. ChatGPT Update: ChatGPT has improved on this, but will not provide evidence nor substantiation to support its claims.
One of the main weaknesses of Large Language Models (LLM) is that their 'chunks' of information (tokens) do not stay together. Therefore, names, places, dates and instances are very rarely correlated accurately. Click here to read an essay that has been generated using GPT-3 that exemplifies this. The GPT series are the most popular LLMs currently; created by OpenAI, they have been publicly available since late 2019. ChatGPT Update: ChatGPT will usually avoid substantiating its claims, so its output rarely will create this problem.
While summarizing and paraphrasing short texts is easily achieved, giving consistent attribution to an author and engaging with metadata (titles, publishing dates, etc.) is nearly impossible for a text generator. The voice used will mimic that of the source text. The caveat to this is if summaries of a text already exist, LLMs can readily automate original-appearing versions (paraphrasing).
Similar to the problem of fake instances, Large Language Models (LLM) will provide unverifiable evidence and claims that are simply untrue. The form will be consistent with linguistic conventions, but the content will be false. Click here to read the original white paper that this infographic is based on. Sections 2.1 - 3.3 have several examples of this idiosyncrasy of AI generated text. ChatGPT Update: This happens much less frequently as ChatGPT rarely attempts to provide evidence unless prompted.
LanguageErrors
Introductions and Conclusions
Idiosyncracies of grammar or sentence structure
short summarized and paraphrased text
Word Choice
Will there be A Technology that can identify AI Generated Text?
Creative Writing Styles
Punctuation Choice
What can not be identified as AI generated...
Concept and Infographic by Ryan Morrison Iceberg Design by Jérémie Boulay
Abstracts Used in academic Publishing
STEMware Inc.
Check out this site, copy.ai (log in required). It contains writing tools in its menu that are specifically aimed at creating introductions, outlines, thesis statements and conclusions.
There are interesting paradoxes that occur in AI generated text, especially from perspective of language education. We often teach which words are most commonly used in which situations, and as a result, these words are used with higher frequency in LLM source texts. Therefore, the the vocabulary of 'standard writing styles' are mirrored in AI generated text, and less frequently used words are omitted during most generative settings. Here is an interesting exploration of this phenomenon from Writefull.
Trying to 'catch' breaches of academic integrity using technology has long been described as an "arms race". However, while tools are important in starting conversations with students about academic integrity, for text generating and transforming technology, they will not be available. As mentioned in this article, a more reasonable approach is to become aware and familiar with this technology. There are several resources throughout 'the iceberg' that can help you reconsider what and how we should be teaching language arts.
If a GPT-3 generator is set to a higher sampler temperature, it will make more 'random' choices. In this case, it might use punctuation like dashes and semi-colons correctly, or occasionally incorrectly. This higher temperature setting also affects the accuracy of content, and the algorithm will make choices that are not just fabricated/ conflated, but it will make claims to facts that are completely false.
This is easily accomplished with a low temperature setting and is one of the most popular functions of LLM. In fact, Speedwriter, a site that specializes its use of GPT-3 for summarizing, paid influencers on TikTok to promote their tool to students in 2021. The hashtag currently has almost 200 million views.
There are a couple of reasons for this:
- Everyone makes grammar mistakes, even AI.
- Depending on the sampling temperature, the errors range from occasional run-on sentences and comma splices (low temperature) to completely unstructured nonsense (high temperature). Everything between these points is possible.
This is a great example of an academic language task that poses few ethical concerns as far as automation; another recent example of this is citation generators. While the ability of LLMs to complete summaries could be problematic in some contexts, this function makes academic publishing more inclusive for English language learners. This site, Writefull (log in required), contains preset tools that assist with academic writing tasks like abstract and title generation.
Because most creative writing is not supported by verifiable facts and rightfully includes fictitious names, almost any creative writing task. In fact, one of the early uses of proto-LLMs was a creative writing experiment from Google. Check it out here. However, meter based poetry like Haiku or Sonnets can not be accurately accomplished.
The short answer is "no", but the long answer is "maybe, a very long time from now, if AI writing becomes truly disruptive, and if we can overcome our current computer processing and energy generation issues." LLMs need to be hosted on very large and power-hungry servers, because they are so massive in size and contain so many parameters. Therefore, one method of identifying if something was being algorithmically generated would be to have an algorithm that mirrors generative algorithm using the same technology. This algorithm would have to be the size of all publicly available LLMs, and simultaneously run checks on all settings, for all publicly available LLMs for every 'detection' action. It is not feasible. Another option is to have an algorithm that checks for the elements mentioned near the tip of the iceberg in this infographic. Such an algorithm would need to verify the existence of sources, claims, quotes and facts. This is much more reasonable, and its utility would stretch beyond identifying AI generated text. I hope you enjoyed this infographic and the whitepaper it was based on. If you'd like to dive deeper, have some ideas or just want to connect with me, you can contact me at ryan@stemware.tech